YesBut: A High-Quality Annotated Multimodal Dataset for evaluating Satire Comprehension capability of Vision-Language Models
Abhilash Nandy, Yash Agarwal, Ashish Patwa, Millon Madhur Das, Aman Bansal, Ankit Raj, Pawan Goyal, Niloy Ganguly
2024-09-23
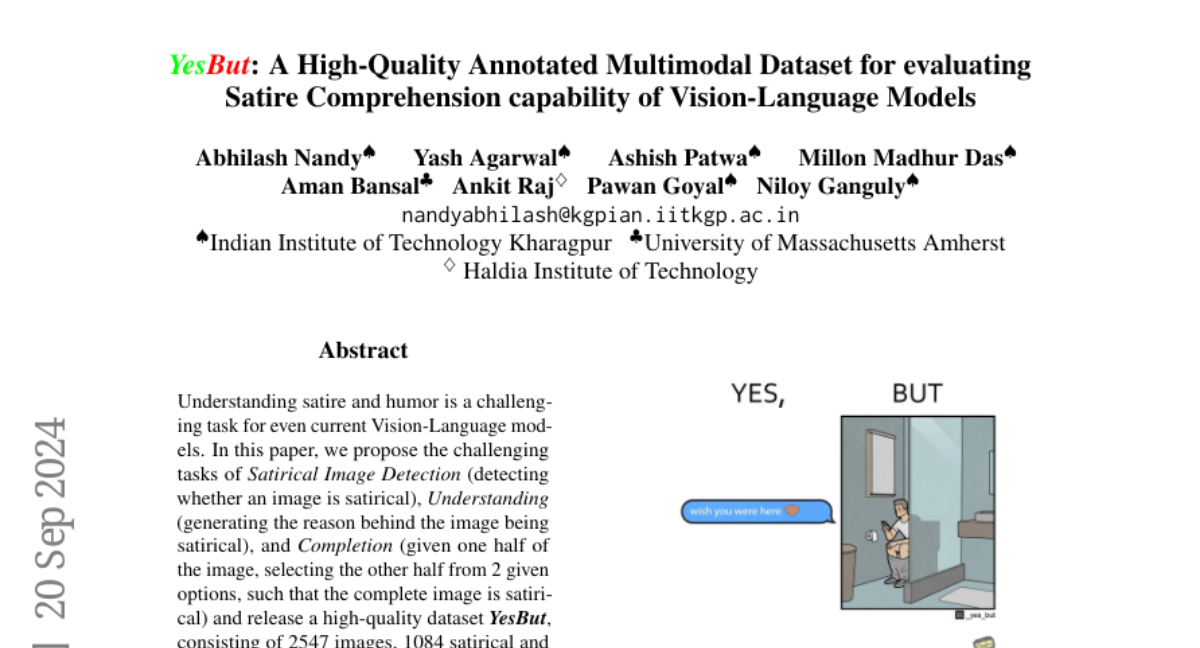
Summary
This paper introduces the YesBut dataset, which is designed to test how well vision-language models can understand satire. It includes a variety of images and tasks to challenge these models in detecting, explaining, and completing satirical content.
What's the problem?
Understanding satire and humor is difficult for even the best AI models. Existing models struggle to accurately identify satirical images, explain why they are funny, and complete them when only part of the image is shown. This lack of understanding limits their effectiveness in tasks that require comprehension of irony and humor.
What's the solution?
The researchers created the YesBut dataset, which consists of 2,547 images—1,084 of which are satirical and 1,463 non-satirical. They developed three main tasks: detecting whether an image is satirical, explaining the humor behind it, and completing a partially visible satirical image by selecting the correct second half from two options. This dataset provides a challenging benchmark for testing the capabilities of vision-language models in recognizing and understanding satire.
Why it matters?
This research is important because it highlights the limitations of current AI models in grasping complex human emotions like humor. By providing a dedicated dataset for evaluating satire comprehension, it encourages further development of models that can better understand and interpret nuanced content, which is crucial for applications in social media, entertainment, and communication.
Abstract
Understanding satire and humor is a challenging task for even current Vision-Language models. In this paper, we propose the challenging tasks of Satirical Image Detection (detecting whether an image is satirical), Understanding (generating the reason behind the image being satirical), and Completion (given one half of the image, selecting the other half from 2 given options, such that the complete image is satirical) and release a high-quality dataset YesBut, consisting of 2547 images, 1084 satirical and 1463 non-satirical, containing different artistic styles, to evaluate those tasks. Each satirical image in the dataset depicts a normal scenario, along with a conflicting scenario which is funny or ironic. Despite the success of current Vision-Language Models on multimodal tasks such as Visual QA and Image Captioning, our benchmarking experiments show that such models perform poorly on the proposed tasks on the YesBut Dataset in Zero-Shot Settings w.r.t both automated as well as human evaluation. Additionally, we release a dataset of 119 real, satirical photographs for further research. The dataset and code are available at https://github.com/abhi1nandy2/yesbut_dataset.