Your Mixture-of-Experts LLM Is Secretly an Embedding Model For Free
Ziyue Li, Tianyi Zhou
2024-10-16
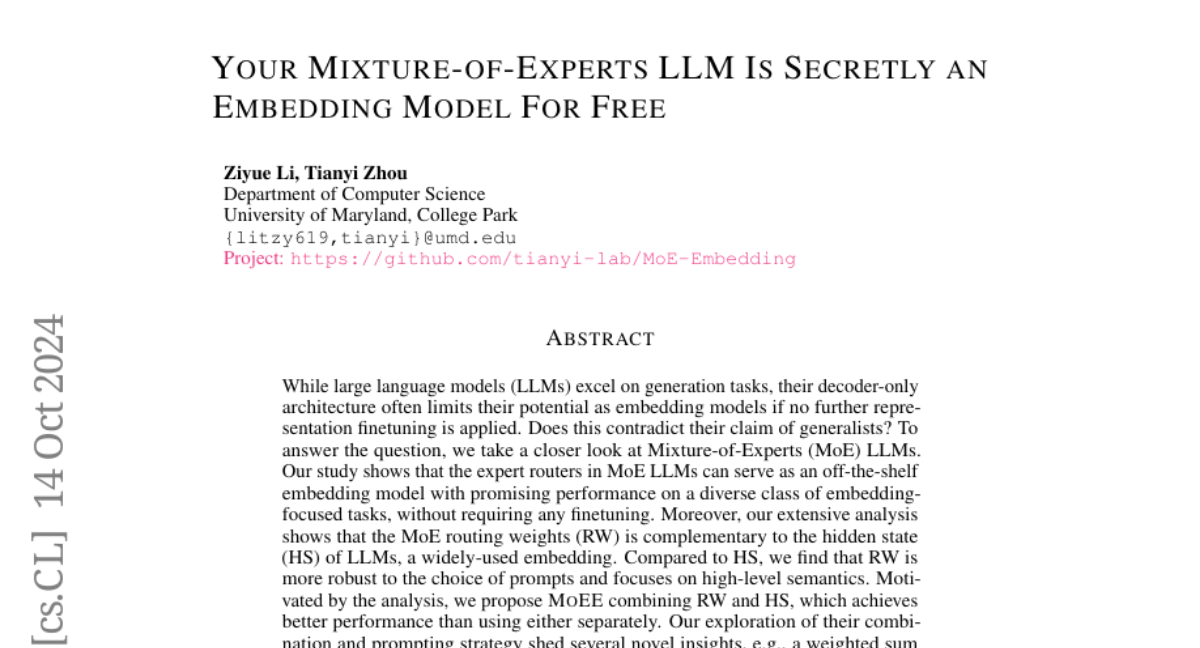
Summary
This paper discusses how Mixture-of-Experts (MoE) large language models (LLMs) can function as effective embedding models without needing extra training, specifically focusing on their internal mechanisms and performance.
What's the problem?
While LLMs are great at generating text, they often struggle to perform well as embedding models, which are used to understand and represent data. This is especially true if they don't receive additional training to adjust their representations. The question arises whether these models can still be considered versatile if they need fine-tuning to work effectively in different tasks.
What's the solution?
The authors explore the internal workings of MoE LLMs and find that the routing mechanisms used to select which 'expert' to use can actually serve as a strong embedding model on their own. They demonstrate that these routing weights are better at maintaining high-level meanings across different prompts compared to traditional hidden states used in LLMs. They propose a new method called MoEE, which combines the strengths of both routing weights and hidden states, leading to improved performance without requiring extra training.
Why it matters?
This research is important because it reveals that MoE LLMs can be more versatile than previously thought, functioning well in various tasks without extensive retraining. This could lead to more efficient AI systems that leverage existing data more effectively, making them useful for a wider range of applications in natural language processing.
Abstract
While large language models (LLMs) excel on generation tasks, their decoder-only architecture often limits their potential as embedding models if no further representation finetuning is applied. Does this contradict their claim of generalists? To answer the question, we take a closer look at Mixture-of-Experts (MoE) LLMs. Our study shows that the expert routers in MoE LLMs can serve as an off-the-shelf embedding model with promising performance on a diverse class of embedding-focused tasks, without requiring any finetuning. Moreover, our extensive analysis shows that the MoE routing weights (RW) is complementary to the hidden state (HS) of LLMs, a widely-used embedding. Compared to HS, we find that RW is more robust to the choice of prompts and focuses on high-level semantics. Motivated by the analysis, we propose MoEE combining RW and HS, which achieves better performance than using either separately. Our exploration of their combination and prompting strategy shed several novel insights, e.g., a weighted sum of RW and HS similarities outperforms the similarity on their concatenation. Our experiments are conducted on 6 embedding tasks with 20 datasets from the Massive Text Embedding Benchmark (MTEB). The results demonstrate the significant improvement brought by MoEE to LLM-based embedding without further finetuning.