ZePo: Zero-Shot Portrait Stylization with Faster Sampling
Jin Liu, Huaibo Huang, Jie Cao, Ran He
2024-08-14
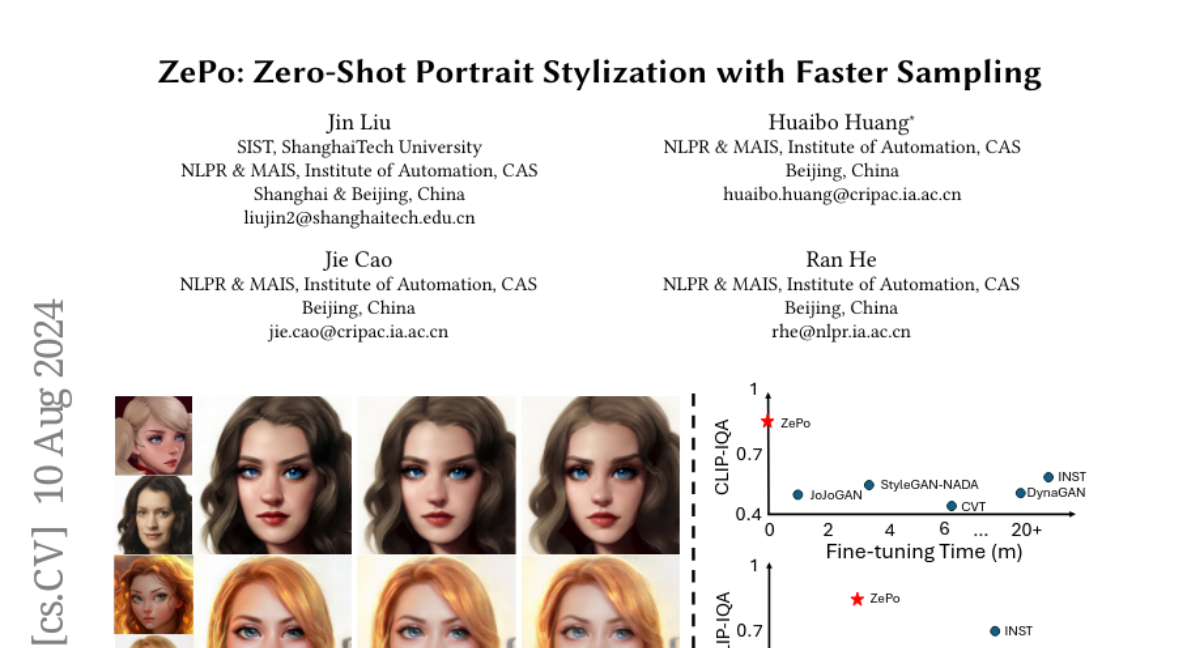
Summary
This paper introduces ZePo, a new method for stylizing portraits using diffusion models that allows for faster image generation without needing to revert images to noise.
What's the problem?
Current methods for stylizing portraits often require additional steps like fine-tuning the model or reverting images back to a noise state, which makes the process slow and complicated. This can be frustrating for artists and developers who want quick results.
What's the solution?
ZePo simplifies the portrait stylization process by using a framework that combines content and style features in just four sampling steps, eliminating the need for inversion. It employs techniques like Latent Consistency Models to extract important features from images and a Style Enhancement Attention Control method to blend these features effectively. This approach not only speeds up the process but also maintains high quality in the stylized images.
Why it matters?
This research is significant because it enhances the efficiency of creating artistic images from portraits, making it easier for creators to produce high-quality stylized artwork quickly. By streamlining the process, ZePo opens up new possibilities for artists and developers in various fields, such as gaming, animation, and digital art.
Abstract
Diffusion-based text-to-image generation models have significantly advanced the field of art content synthesis. However, current portrait stylization methods generally require either model fine-tuning based on examples or the employment of DDIM Inversion to revert images to noise space, both of which substantially decelerate the image generation process. To overcome these limitations, this paper presents an inversion-free portrait stylization framework based on diffusion models that accomplishes content and style feature fusion in merely four sampling steps. We observed that Latent Consistency Models employing consistency distillation can effectively extract representative Consistency Features from noisy images. To blend the Consistency Features extracted from both content and style images, we introduce a Style Enhancement Attention Control technique that meticulously merges content and style features within the attention space of the target image. Moreover, we propose a feature merging strategy to amalgamate redundant features in Consistency Features, thereby reducing the computational load of attention control. Extensive experiments have validated the effectiveness of our proposed framework in enhancing stylization efficiency and fidelity. The code is available at https://github.com/liujin112/ZePo.