Zero-shot Model-based Reinforcement Learning using Large Language Models
Abdelhakim Benechehab, Youssef Attia El Hili, Ambroise Odonnat, Oussama Zekri, Albert Thomas, Giuseppe Paolo, Maurizio Filippone, Ievgen Redko, Balázs Kégl
2024-10-22
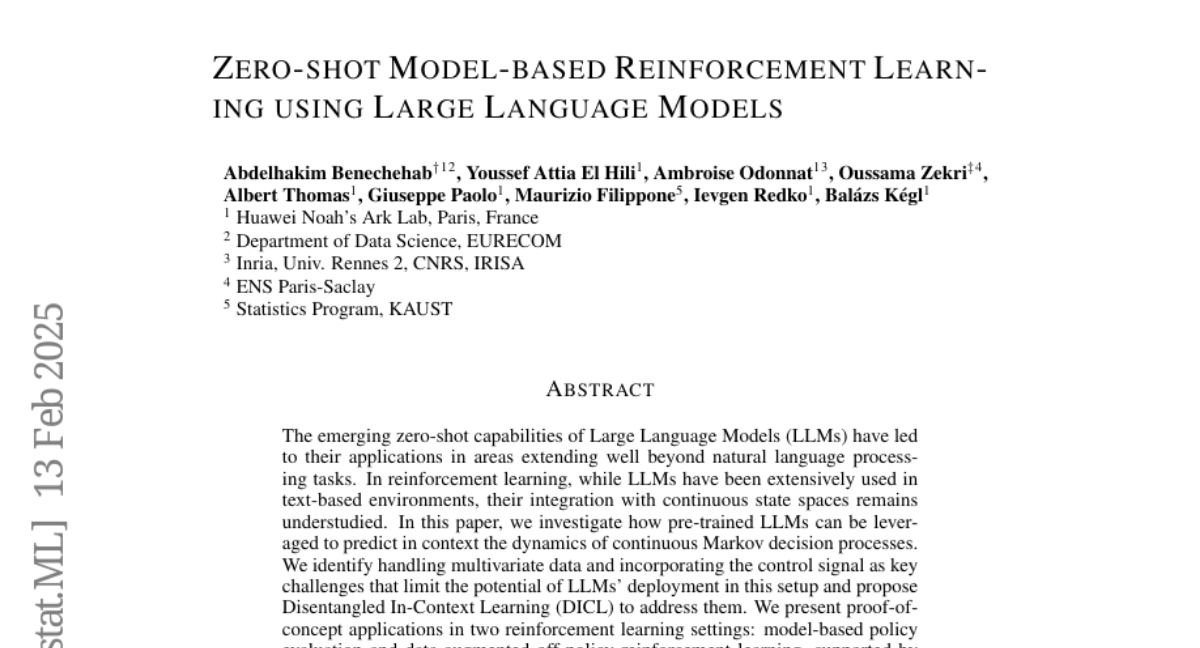
Summary
This paper discusses how to use large language models (LLMs) in a new way for reinforcement learning, specifically focusing on a method called zero-shot model-based reinforcement learning.
What's the problem?
While LLMs have been successful in understanding and generating text, their use in reinforcement learning (RL) tasks that involve continuous data (like movement or actions over time) is still not well explored. Most existing methods focus on text-based environments, leaving a gap in how these models can be applied to more complex situations where they need to predict outcomes based on continuous inputs.
What's the solution?
To address this issue, the authors propose a method called Disentangled In-Context Learning (DICL), which helps LLMs better understand and predict the dynamics of continuous Markov decision processes. They identify challenges such as handling complex data and incorporating control signals and provide solutions through their new framework. The authors conducted experiments in two RL settings: model-based policy evaluation and data-augmented off-policy reinforcement learning, showing that their approach leads to more accurate predictions and better performance.
Why it matters?
This research is important because it expands the capabilities of LLMs beyond traditional text tasks, allowing them to be used effectively in more complex reinforcement learning scenarios. By improving how these models learn from continuous data, the findings could lead to advancements in AI applications like robotics, gaming, and autonomous systems, where understanding dynamic environments is crucial.
Abstract
The emerging zero-shot capabilities of Large Language Models (LLMs) have led to their applications in areas extending well beyond natural language processing tasks. In reinforcement learning, while LLMs have been extensively used in text-based environments, their integration with continuous state spaces remains understudied. In this paper, we investigate how pre-trained LLMs can be leveraged to predict in context the dynamics of continuous Markov decision processes. We identify handling multivariate data and incorporating the control signal as key challenges that limit the potential of LLMs' deployment in this setup and propose Disentangled In-Context Learning (DICL) to address them. We present proof-of-concept applications in two reinforcement learning settings: model-based policy evaluation and data-augmented off-policy reinforcement learning, supported by theoretical analysis of the proposed methods. Our experiments further demonstrate that our approach produces well-calibrated uncertainty estimates. We release the code at https://github.com/abenechehab/dicl.