ZeroComp: Zero-shot Object Compositing from Image Intrinsics via Diffusion
Zitian Zhang, Frédéric Fortier-Chouinard, Mathieu Garon, Anand Bhattad, Jean-François Lalonde
2024-10-14
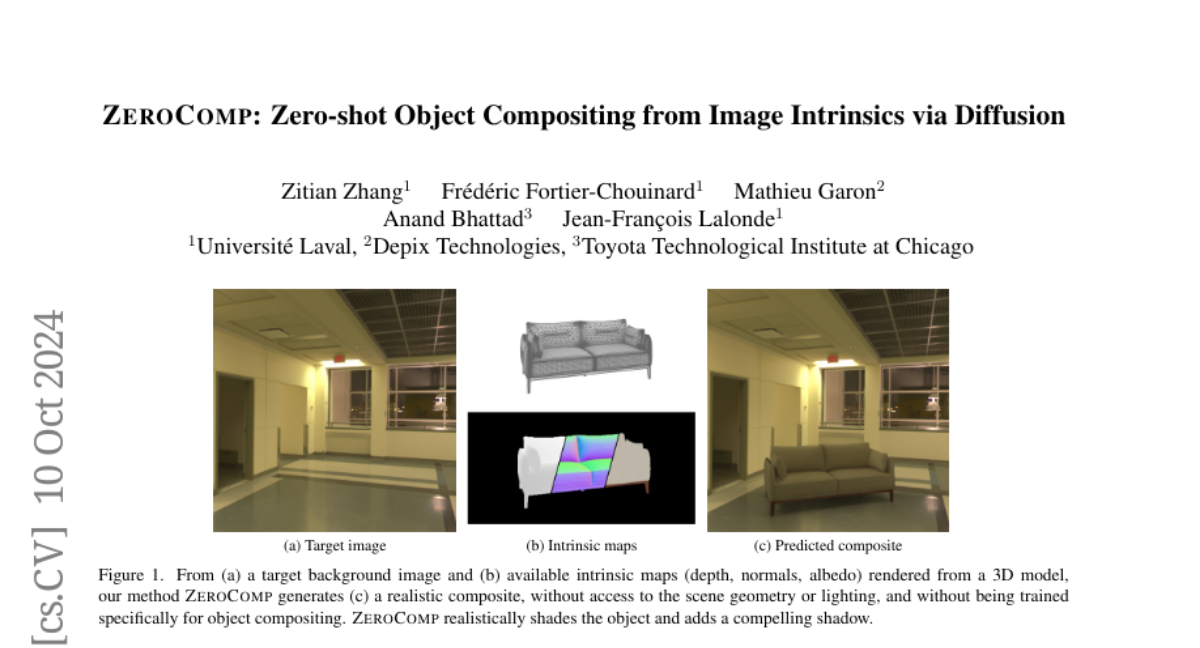
Summary
This paper introduces ZeroComp, a new method for combining 3D objects into images without needing specific training images that show how the objects should look in different scenes.
What's the problem?
Creating realistic 3D objects and placing them into images can be difficult because traditional methods often require paired images—one showing the scene without the object and one with it. This means you need a lot of specific training data, which is hard to get.
What's the solution?
ZeroComp uses a technique that relies on intrinsic images, which capture important details like geometry and color, instead of needing those paired images. It employs a model called ControlNet to help understand these intrinsic images and combines it with a Stable Diffusion model to generate realistic images. This way, it can insert virtual 3D objects into various scenes while adjusting the lighting to make everything look natural.
Why it matters?
This research is significant because it allows for more flexible and efficient ways to create realistic images with 3D objects, even when using limited training data. This could be useful in fields like gaming, virtual reality, and film production, where adding realistic 3D elements to scenes is important.
Abstract
We present ZeroComp, an effective zero-shot 3D object compositing approach that does not require paired composite-scene images during training. Our method leverages ControlNet to condition from intrinsic images and combines it with a Stable Diffusion model to utilize its scene priors, together operating as an effective rendering engine. During training, ZeroComp uses intrinsic images based on geometry, albedo, and masked shading, all without the need for paired images of scenes with and without composite objects. Once trained, it seamlessly integrates virtual 3D objects into scenes, adjusting shading to create realistic composites. We developed a high-quality evaluation dataset and demonstrate that ZeroComp outperforms methods using explicit lighting estimations and generative techniques in quantitative and human perception benchmarks. Additionally, ZeroComp extends to real and outdoor image compositing, even when trained solely on synthetic indoor data, showcasing its effectiveness in image compositing.