ZIP-FIT: Embedding-Free Data Selection via Compression-Based Alignment
Elyas Obbad, Iddah Mlauzi, Brando Miranda, Rylan Schaeffer, Kamal Obbad, Suhana Bedi, Sanmi Koyejo
2024-10-25
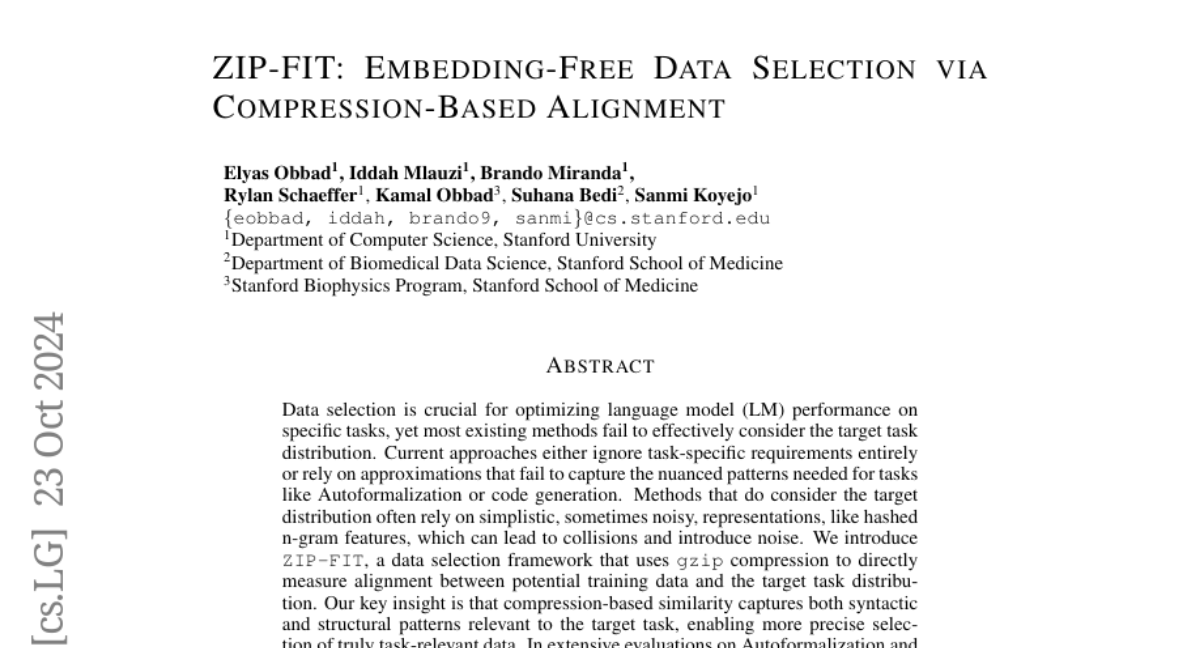
Summary
This paper introduces ZIP-FIT, a new method for selecting data that improves the performance of language models by using gzip compression to align training data with specific tasks.
What's the problem?
Choosing the right data for training language models is crucial for their success on specific tasks, but many existing methods either ignore the specific needs of these tasks or use overly simplistic techniques that can introduce errors. This can lead to poor performance, especially in complex tasks like Autoformalization or code generation.
What's the solution?
The authors propose ZIP-FIT, which uses gzip compression to measure how well potential training data aligns with the target task. This method allows for more precise selection of relevant data, leading to better training outcomes. In tests on tasks like Autoformalization and Python code generation, models trained with ZIP-FIT-selected data performed significantly better and learned faster than those trained with other methods. The results showed that smaller, well-chosen datasets can outperform larger, less relevant ones.
Why it matters?
This research is important because it demonstrates that focusing on the quality and relevance of training data can greatly enhance the efficiency and effectiveness of language models. By showing that better task alignment leads to improved learning, ZIP-FIT provides valuable insights for developing more effective AI systems in various applications.
Abstract
Data selection is crucial for optimizing language model (LM) performance on specific tasks, yet most existing methods fail to effectively consider the target task distribution. Current approaches either ignore task-specific requirements entirely or rely on approximations that fail to capture the nuanced patterns needed for tasks like Autoformalization or code generation. Methods that do consider the target distribution often rely on simplistic, sometimes noisy, representations, like hashed n-gram features, which can lead to collisions and introduce noise. We introduce ZIP-FIT, a data selection framework that uses gzip compression to directly measure alignment between potential training data and the target task distribution. In extensive evaluations on Autoformalization and Python code generation, ZIP-FIT significantly outperforms leading baselines like DSIR and D4. Models trained on ZIP-FIT-selected data achieve their lowest cross-entropy loss up to 85.1\% faster than baselines, demonstrating that better task alignment leads to more efficient learning. In addition, ZIP-FIT performs selection up to 65.8\% faster than DSIR and two orders of magnitude faster than D4. Notably, ZIP-FIT shows that smaller, well-aligned datasets often outperform larger but less targeted ones, demonstrating that a small amount of higher quality data is superior to a large amount of lower quality data. Our results imply that task-aware data selection is crucial for efficient domain adaptation, and that compression offers a principled way to measure task alignment. By showing that targeted data selection can dramatically improve task-specific performance, our work provides new insights into the relationship between data quality, task alignment, and model learning efficiency.