SAMPart3D: Segment Any Part in 3D Objects
Yunhan Yang, Yukun Huang, Yuan-Chen Guo, Liangjun Lu, Xiaoyang Wu, Edmund Y. Lam, Yan-Pei Cao, Xihui Liu
2024-11-13
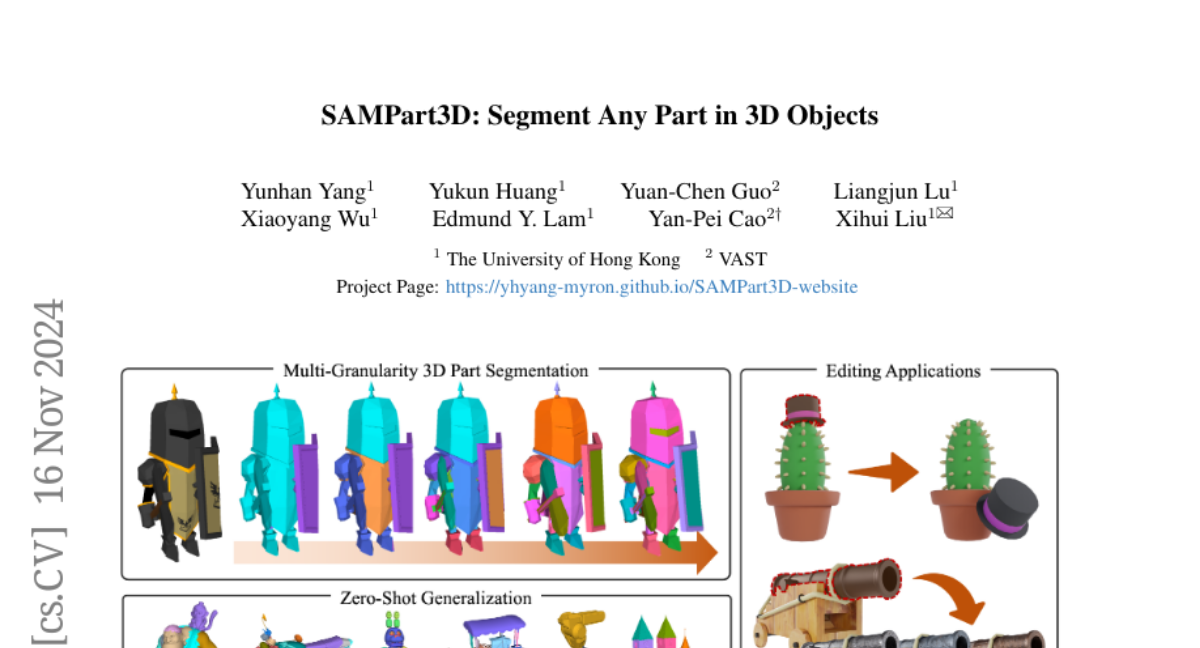
Summary
This paper talks about SAMPart3D, a new framework designed to segment 3D objects into different parts without needing predefined labels. It aims to improve how we understand and manipulate 3D shapes in various applications like robotics and 3D editing.
What's the problem?
The problem is that current methods for segmenting 3D objects often rely on large amounts of labeled data and specific text prompts, which can limit their ability to work with new, unlabeled datasets. This makes it hard to scale these methods to handle a wide variety of 3D objects, especially complex ones.
What's the solution?
The authors introduce SAMPart3D, which uses a different approach by not requiring predefined labels. Instead, it employs vision foundation models that can learn from large amounts of unlabeled 3D data. This allows the framework to segment parts of 3D objects at different levels of detail. They also developed a new benchmark dataset to help test the effectiveness of their method. By doing extensive experiments, they showed that SAMPart3D performs much better than previous methods in segmenting complex 3D objects.
Why it matters?
This research is important because it opens up new possibilities for working with 3D objects in technology and design. By improving how we can segment and understand 3D shapes without needing a lot of labeled data, it can lead to advancements in fields like robotics, game design, and virtual reality.
Abstract
3D part segmentation is a crucial and challenging task in 3D perception, playing a vital role in applications such as robotics, 3D generation, and 3D editing. Recent methods harness the powerful Vision Language Models (VLMs) for 2D-to-3D knowledge distillation, achieving zero-shot 3D part segmentation. However, these methods are limited by their reliance on text prompts, which restricts the scalability to large-scale unlabeled datasets and the flexibility in handling part ambiguities. In this work, we introduce SAMPart3D, a scalable zero-shot 3D part segmentation framework that segments any 3D object into semantic parts at multiple granularities, without requiring predefined part label sets as text prompts. For scalability, we use text-agnostic vision foundation models to distill a 3D feature extraction backbone, allowing scaling to large unlabeled 3D datasets to learn rich 3D priors. For flexibility, we distill scale-conditioned part-aware 3D features for 3D part segmentation at multiple granularities. Once the segmented parts are obtained from the scale-conditioned part-aware 3D features, we use VLMs to assign semantic labels to each part based on the multi-view renderings. Compared to previous methods, our SAMPart3D can scale to the recent large-scale 3D object dataset Objaverse and handle complex, non-ordinary objects. Additionally, we contribute a new 3D part segmentation benchmark to address the lack of diversity and complexity of objects and parts in existing benchmarks. Experiments show that our SAMPart3D significantly outperforms existing zero-shot 3D part segmentation methods, and can facilitate various applications such as part-level editing and interactive segmentation.